Articles
Articles and stories on timely topics and trends across a variety of industries.
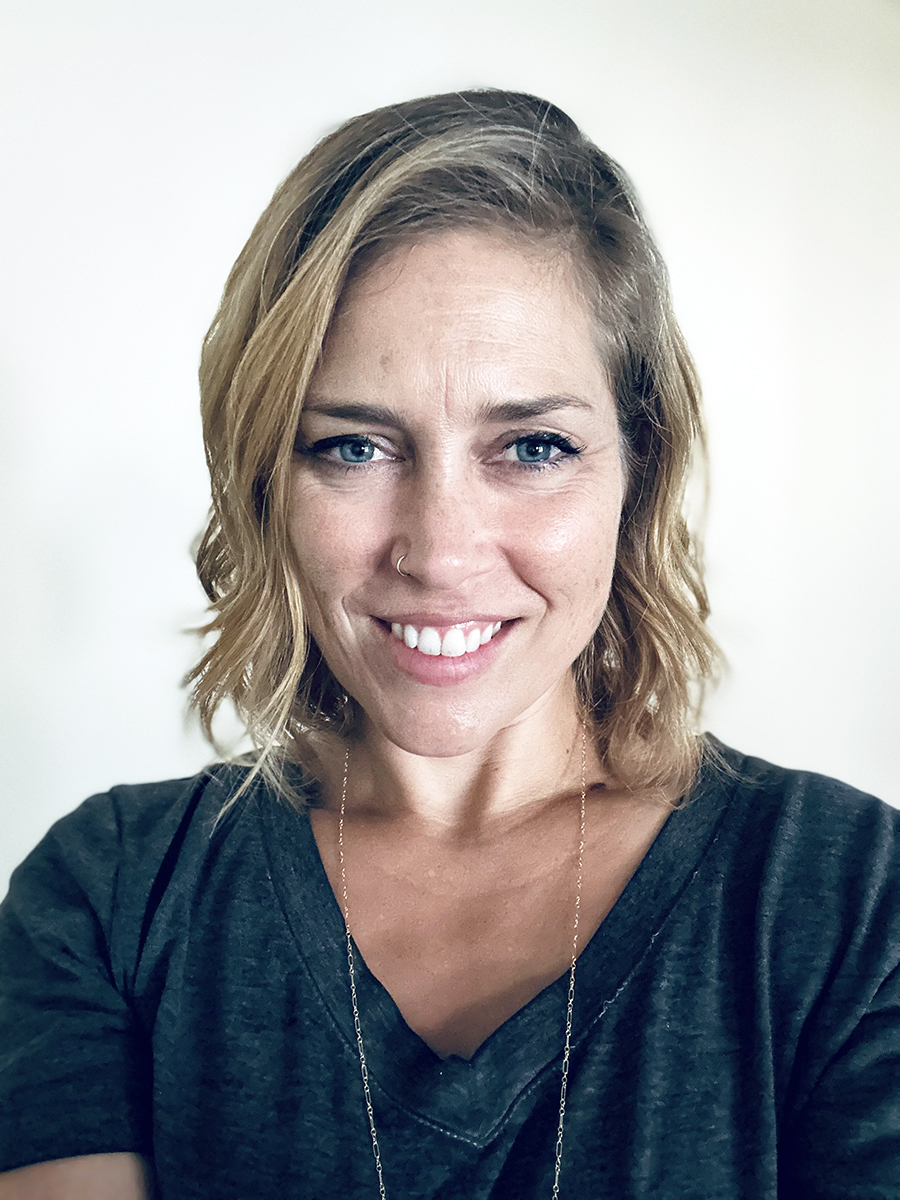
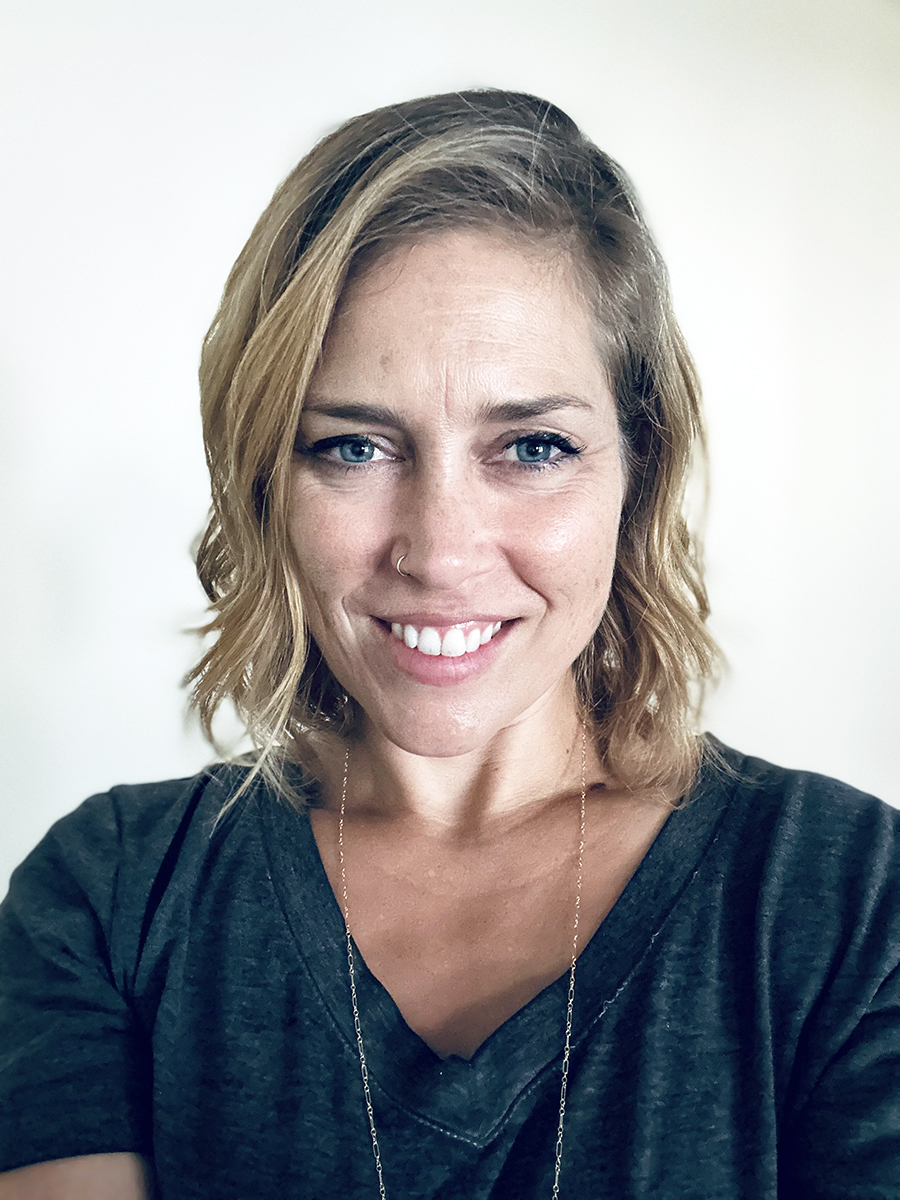
Making Meaningful Work a Profitable Business
Allie Boman, Editing certificate
Read time – 4 minutes
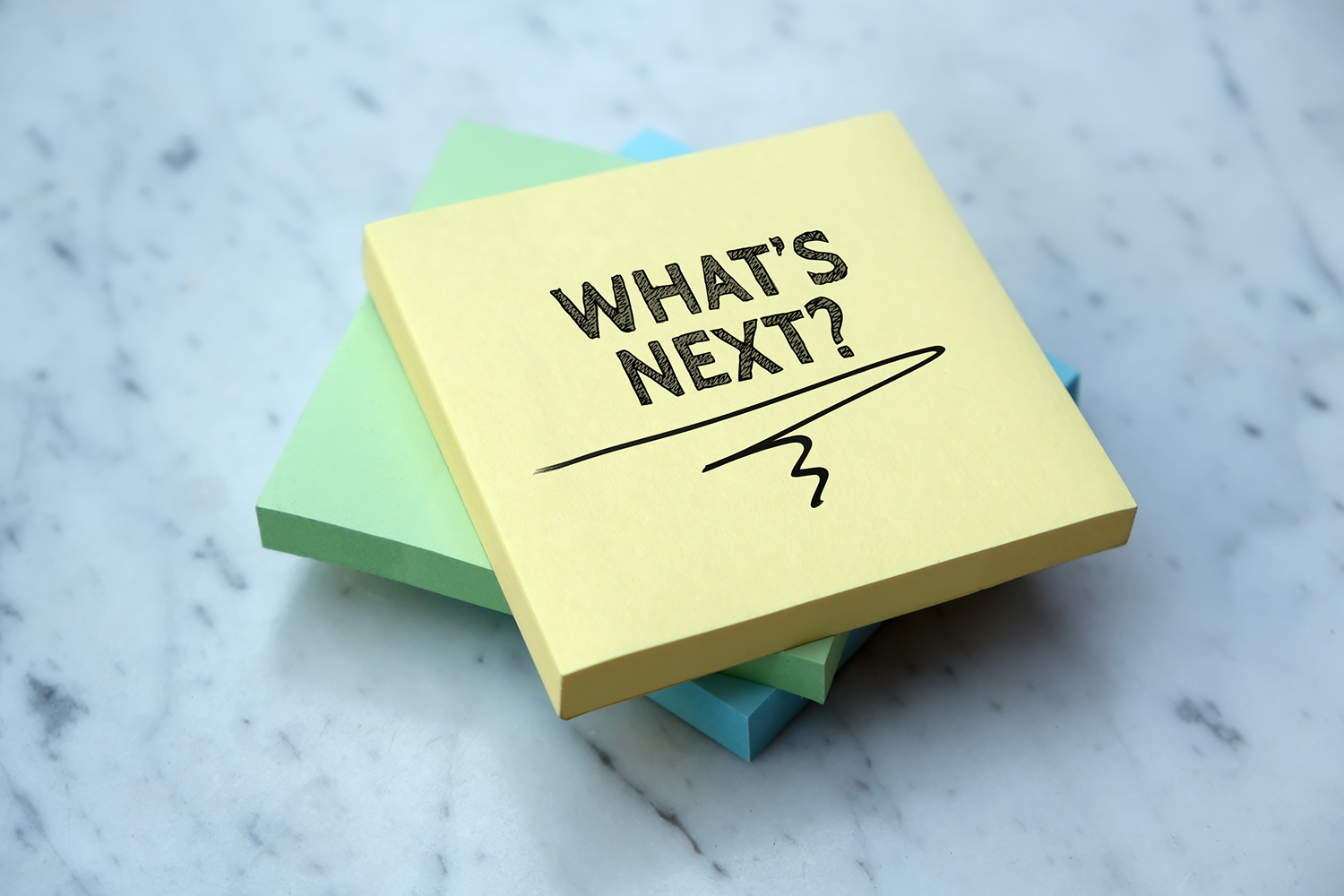
When Expertise Isn’t Enough
How professionals are rewriting their stories through medical communications
Read time – 6 minutes
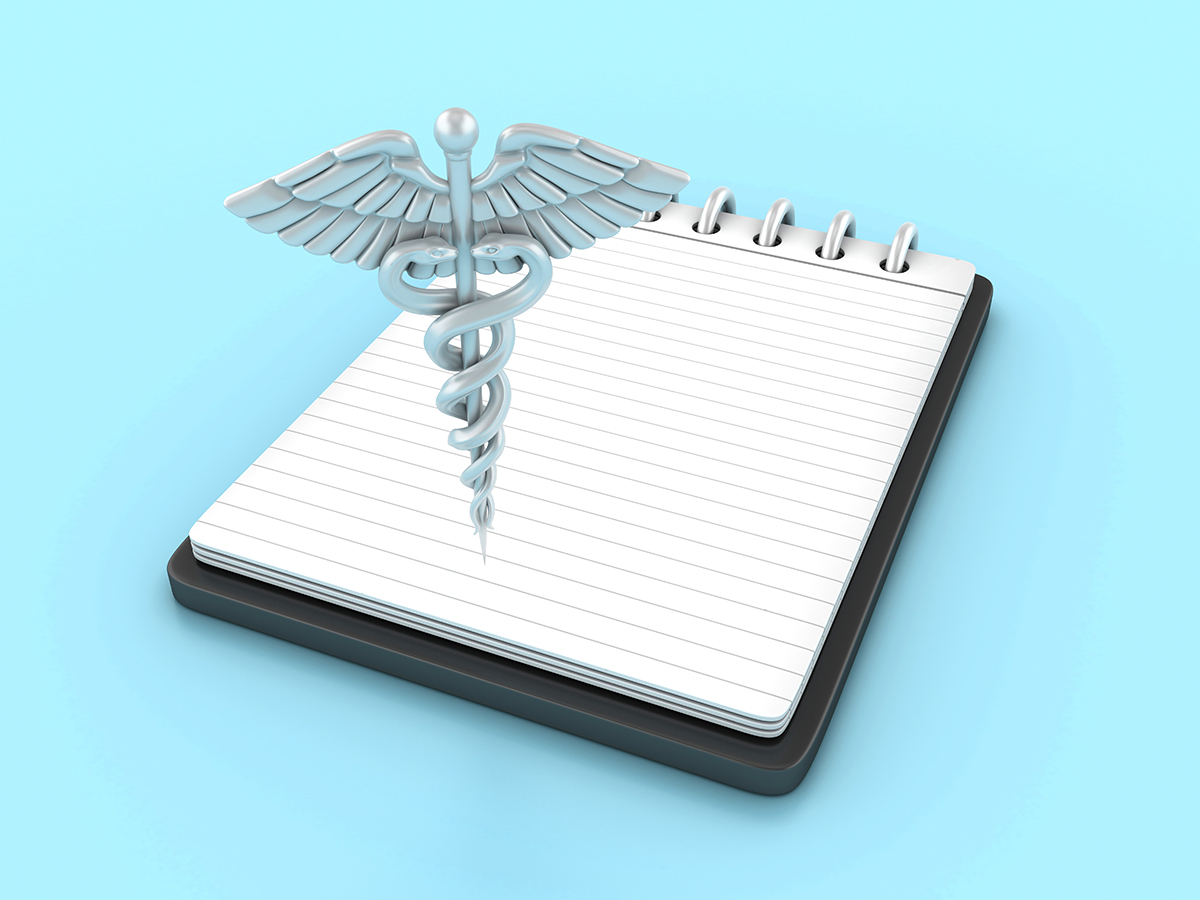
The Science of Translation: How Medical Communicators Are Reshaping Healthcare
Writers have to synthesize increasingly complex material for a range of audiences by acting as bridges between scientific innovation and public understanding.
Read time – 7 minutes
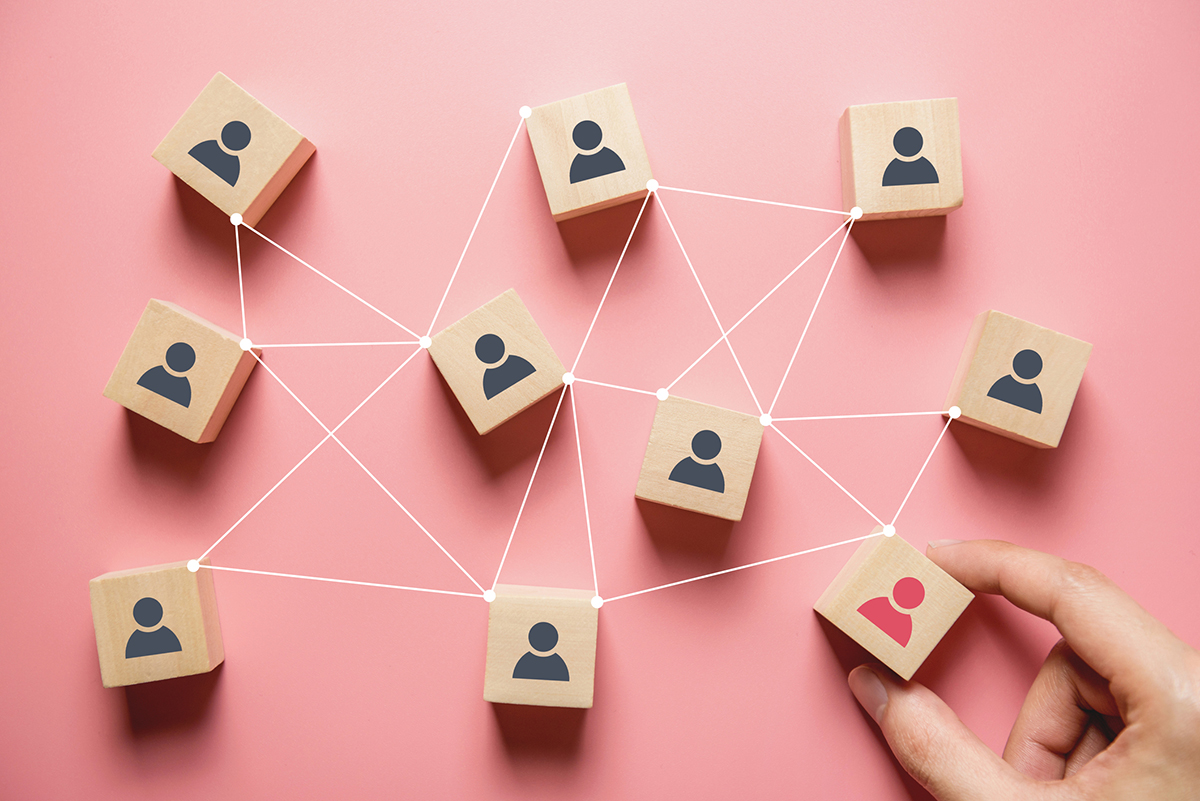
The Essential Role of Specialized Executive Education in Today’s C-Suite
Gaining diverse skills that span multiple functions requires training programs designed to quickly adapt to the evolving demands of the C-Suite.
Read time – 6 minutes
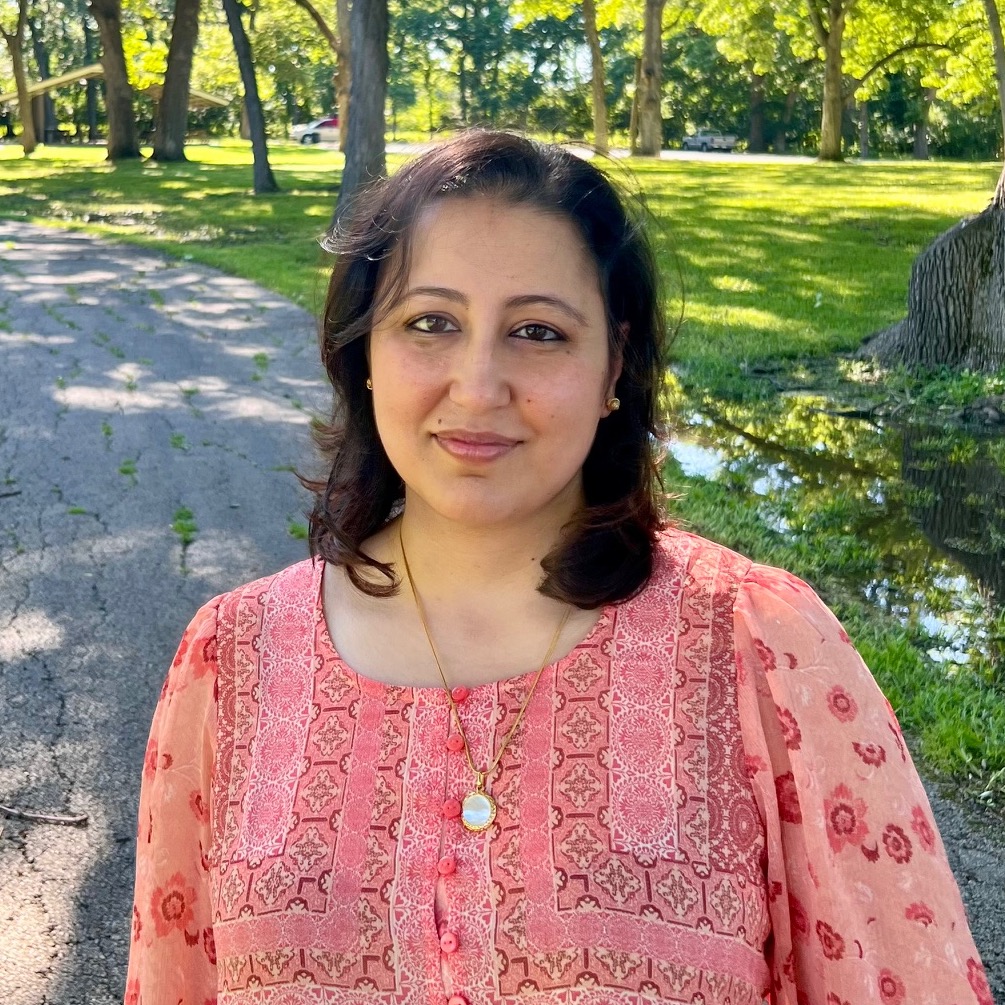
A Psychiatrist Forges a Career Path Through Medical Writing
Vishwani Sahai, MD, certificate in Medical Writing and Editing
Read time – 3 minutes
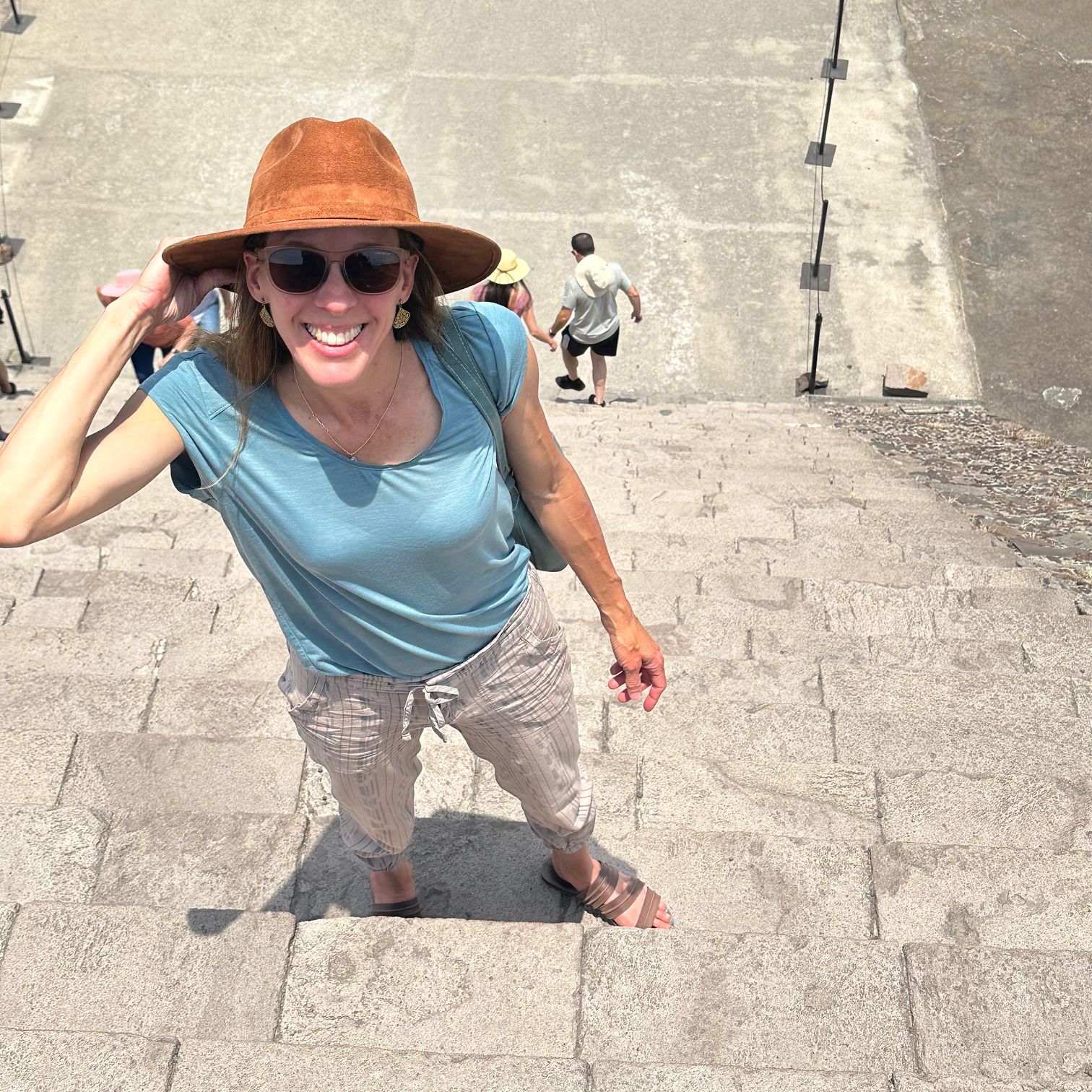
From Science Writer to Medical Communicator
DeLene Beeland, instructor, certificate in Medical Writing and Editing
Read time – 4 minutes
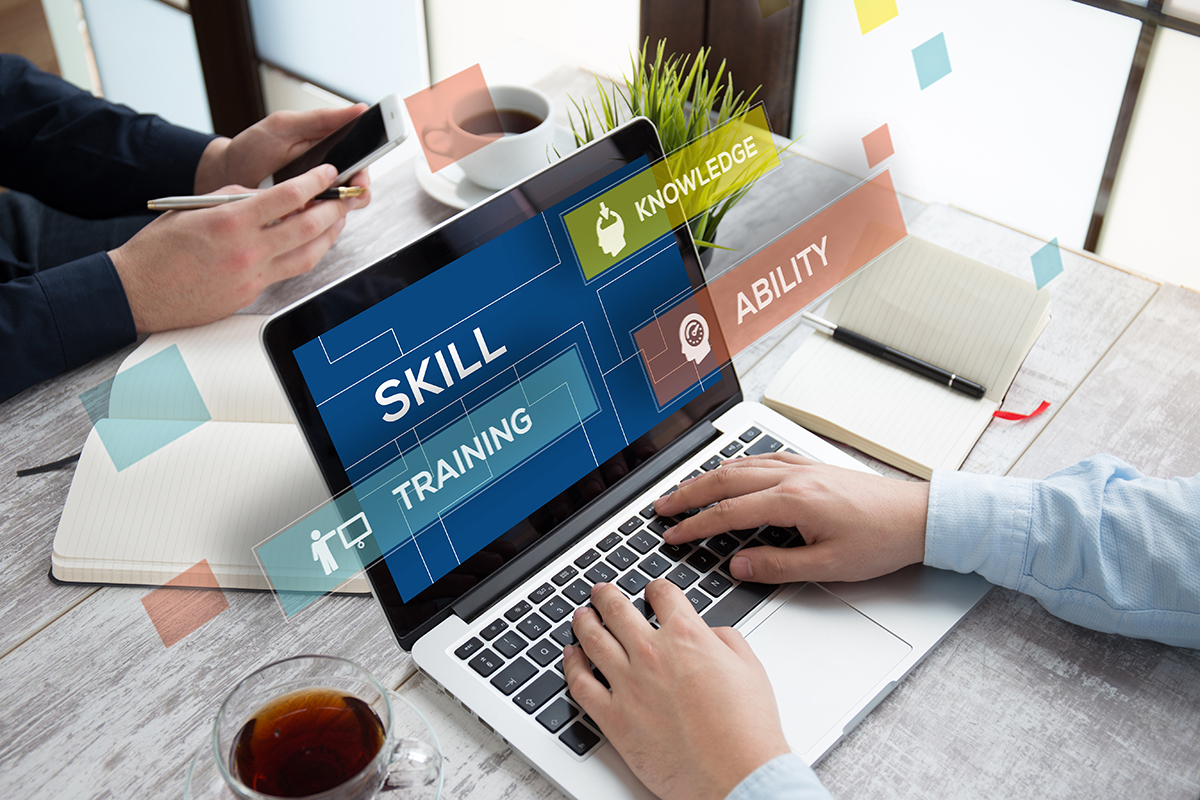
Professional Development Bootcamps: Connecting Skills to Opportunity
As traditional career paths fragment and skill lifecycles accelerate, professional development bootcamps have shifted decisively from niche experiments to powerful drivers of professional reinvention.
Read time – 5 minutes
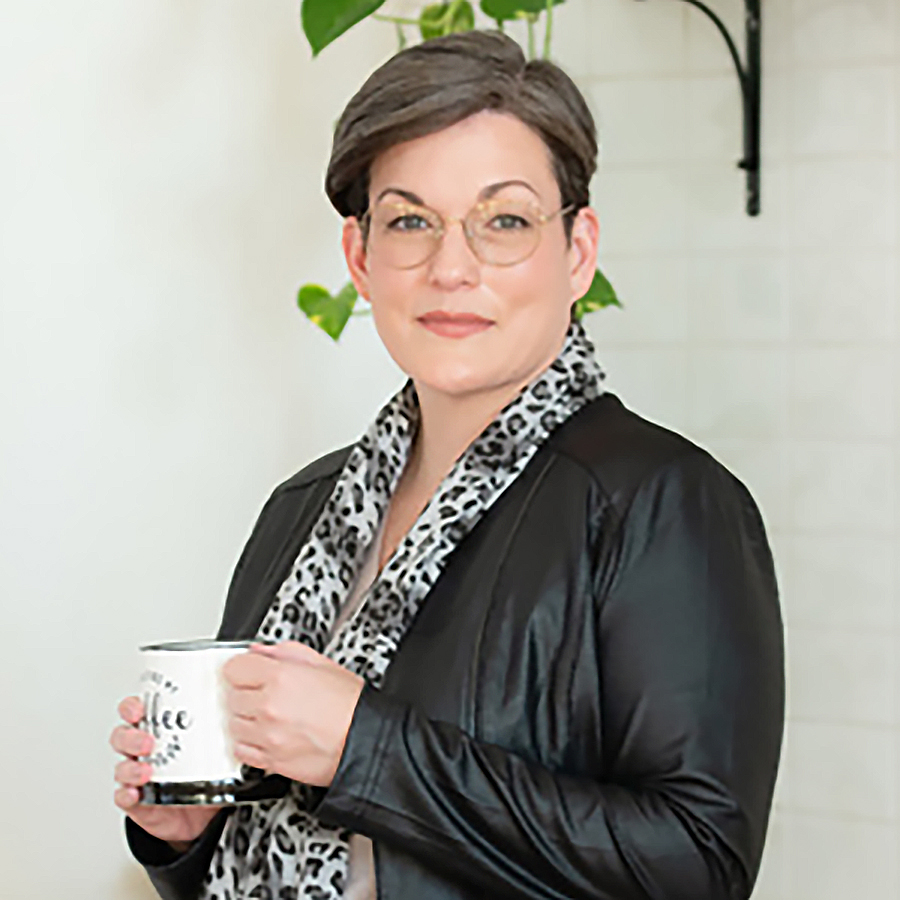
Bridging Medicine and Marketing Through Scientific Storytelling
Holly Hagan, instructor, Healthcare Communications certificate
Read time – 3 minutes
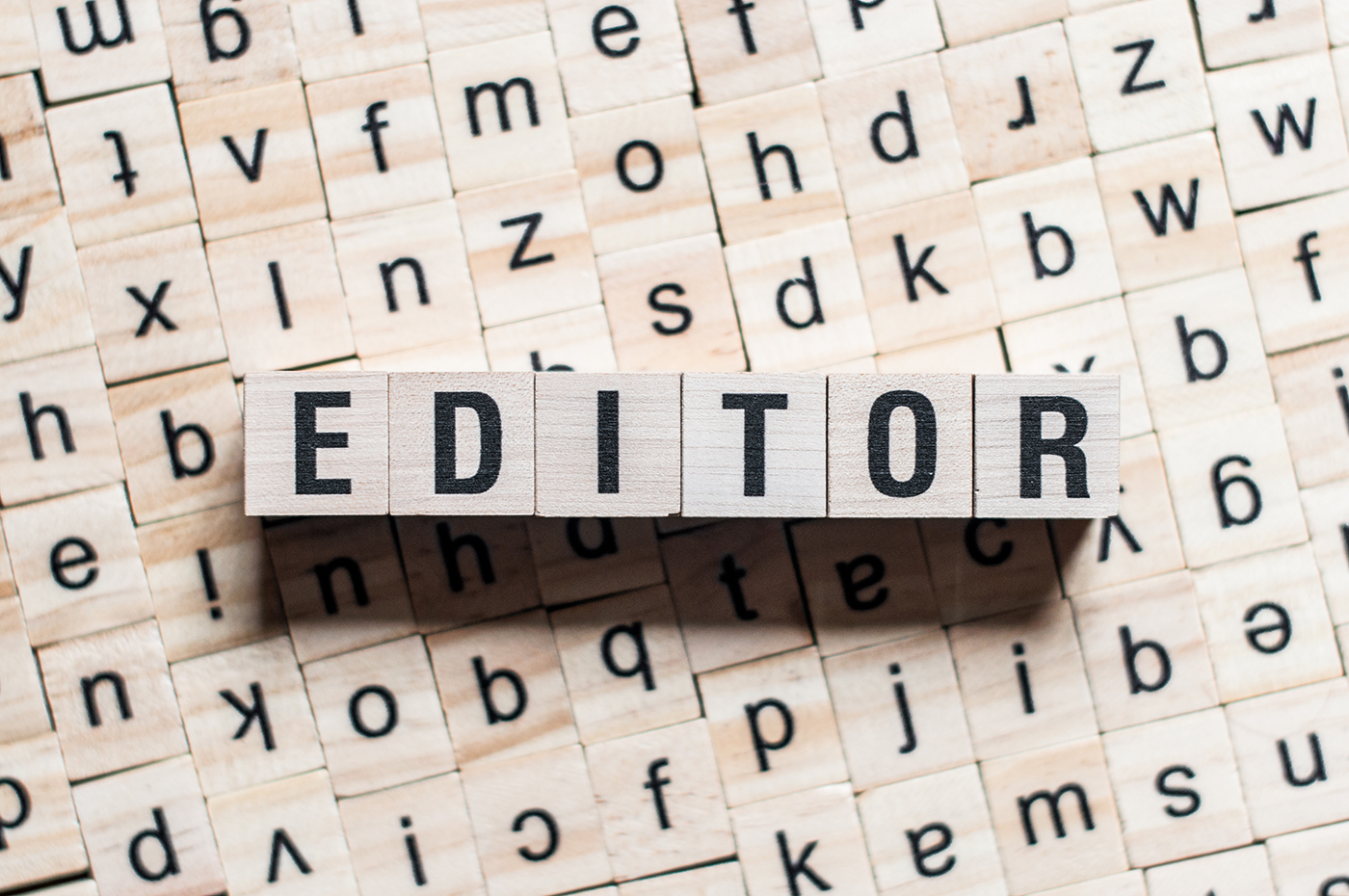
Editing Excellence, One Course at a Time
UChicago delivers targeted courses focused on essential niche skills and specialized editing expertise.
Read time – 5 minutes
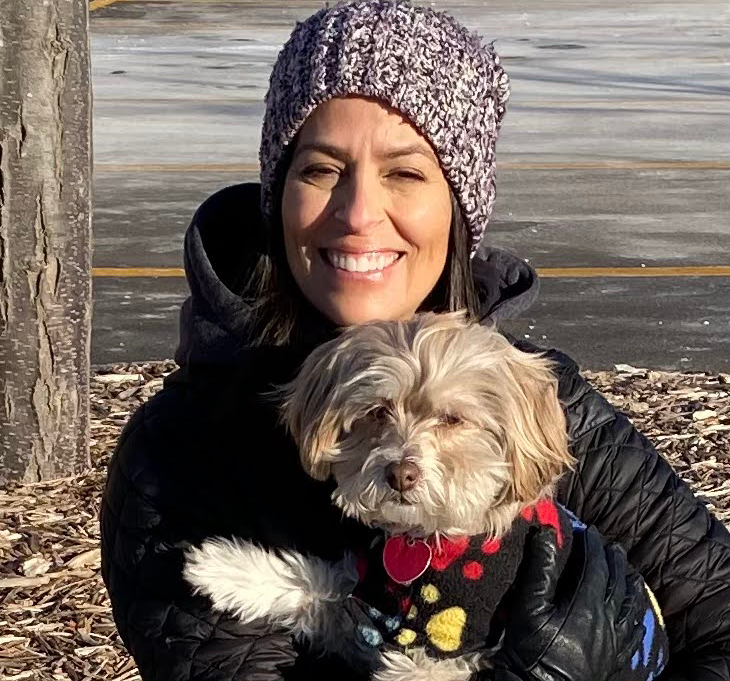
A Veterinarian’s Journey to Medical Writing
Kathy Adamson, MDV, certificate in Medical Writing and Editing
Read time – 3 minutes