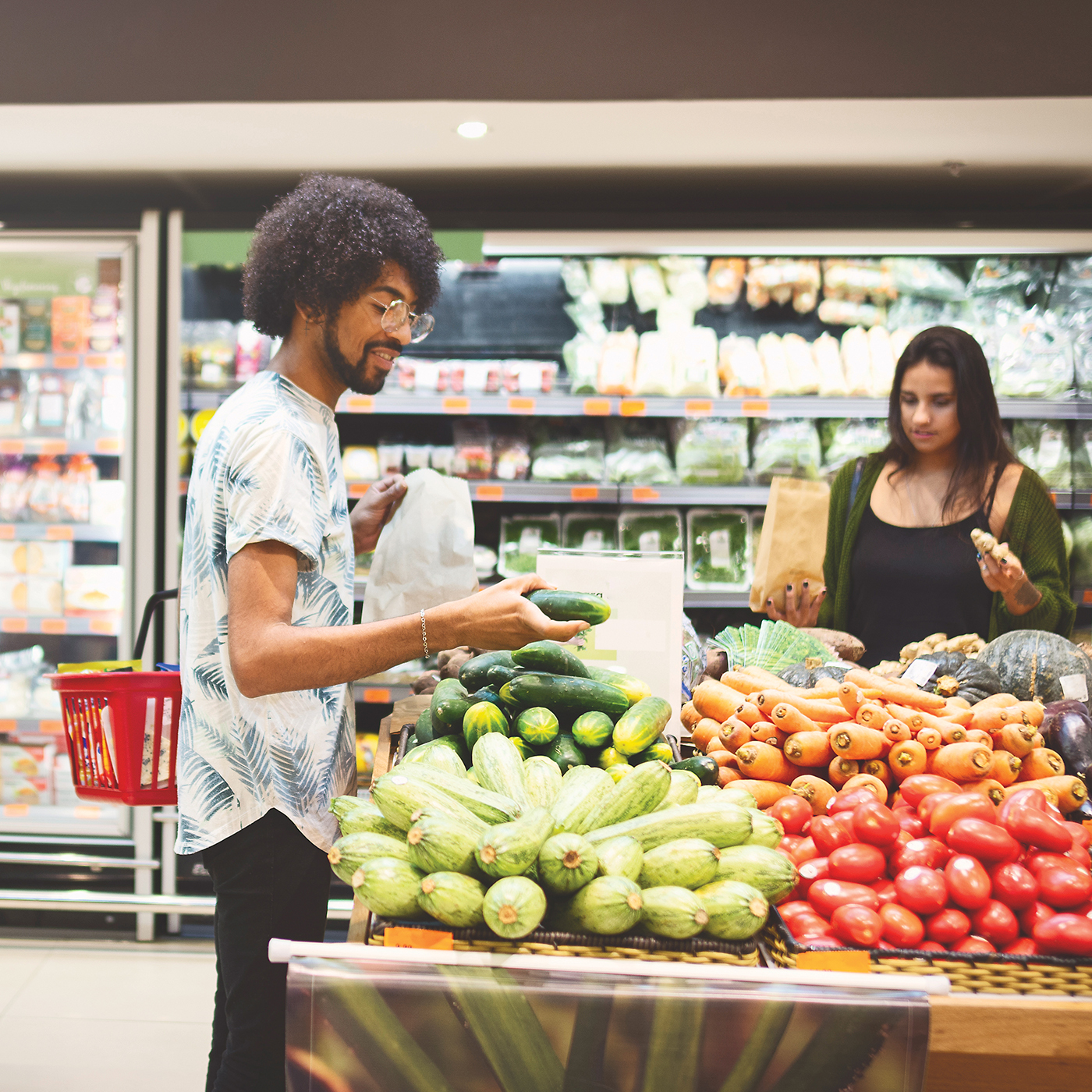
What's in Your Cart?
A pandemic inspires solutions for smarter and healthier online shopping
Challenge
Online grocery shoppers choose poor nutrition quality products since product labels provide difficult-to-understand and complex nutrition content.
Solution
An online recommendation system can help customers select healthier food alternatives by providing them with targeted food recommendations based on the overall nutrition of food items.
Results
Nutrition scores provide consumers with an easy-to-understand rating system for food while they shop online, offer online food retailers with additional revenue from premium food sales, and improve the overall health of the populace.
During the coronavirus pandemic, 79% of consumers bought groceries online, a jump from 19% the year prior, according to Inmar Intelligence. At the same time, findings published in the journal Obesity showed that, out of comfort or convenience, the lockdowns resulted in more people turning to junk food.
While pursuing his Master of Science in Analytics, Yiyan (James) Wan noticed his pandemic eating habits declining, which helped inspire the “Best-in-Show” capstone project he worked on with Reshma Patil and Rameez Jafri. Could they build a system to recommend healthier food alternatives to customers who grocery shop online?
The team wanted to develop an online recommendation system to help customers select healthier food alternatives by providing them with targeted food recommendations based on the overall nutrition of food items. To that end, they developed a new model to compute the nutrient content of food items based on ingredients and processing methods, incorporating methodologies suggested in academic literature in health and nutrition. They used the nutrition score in comparing three data science methods based on machine learning models to present online shoppers with healthier food options tailored to their shopping history.
An early background in nutrition tools
Patil, now a senior data scientist at CVS Health, was motivated by her background in healthcare. In 2015, she worked for a Singapore-based healthcare company called Holmusk. “Holmusk was building an in-house app which has diabetes patients track their food and how much time they have to spend on exercise,” says Patil. “I realized there is a huge gap in how consumers can supervise whether they are buying healthier food or not at the time of purchase.”
The team began with data description and analysis, using visualization techniques and Python programming to clean the store data and make it consistent. For the next step, they had to decide on the metrics they would use to assess the data.“What is the real-life implication when we define health in one way as opposed to another?” says Patil. From there, they needed to consider what type of model to build, starting with a classic model based on market-based analysis, a technique used by large retailers to uncover associations between items.
Going solo
Ultimately, the team incorporated methodologies they gleaned from health and nutritional studies. By comparing three data science methods based on machine learning models, they could recommend alternate healthier food options to people while shopping online, based on their shopping history, nutrition scores, and prices of food items. But a significant challenge at first was a lack of data. Most capstone projects pair students with a business in need of data science to solve a challenge, but the team worked independently. “Other [capstone] teams who partner with businesses or industry leaders are given the data up front. We had to collect and consolidate what we needed by ourselves,” says Wan. On top of the publicly available grocery transactional data, the team needed to incorporate price and nutrition data.
Ultimately, the team utilized an API to scrape 60,000 independent food prices, but even their eventual automated process had its roadblocks. “Due to the daily limit on the API, we had to create a whole set of different usernames and passwords,” says Wan. But the challenge was a learning experience.
“I got to know about the importance of defining your metric clearly, which made modeling more accurate,” says Patil. “Also, we had to build our domain knowledge which played a crucial role in this project. That was something that surprised me.”
Tools and teams
Wan credits the UChicago programming, data mining and statistics courses with giving the team the tools they needed to see the project through. “The courses basically open the doors of data science to you. And then, once we have familiarity with the models, we can start exploring all the different ways you can apply them.”
They also received help from their supervisor, Anil Chaturvedi. “He has a lot of experience in marketing analytics. One of our steps was to segment our customers: he provided a lot of insights on what kind of features would be useful for our model training and feature engineering afterwards,” says Wan, who also says his team’s chemistry played a role in its success. “We all really bonded and got aligned on goals. For a project this long—nine months—you need someone who can keep motivating, inspiring and cheering you up.”
The team foresees that consumers, online food retailers, and the government will benefit from using their comprehensive nutrition measures. “I think many people during the pandemic were looking for ways to eat healthier,” says Wan. “I know I was. That’s why it seemed like the perfect time to build a tool that clearly shows the way to healthier eating.”